Modelling the transmission of infectious disease
Mathematical models of disease transmission can be used to estimate the potential impact of public health responses to infectious diseases. Recently (7 April) some details of the particular model that is being used as the basis for the decisions of Australian governments on the COVID-19 crisis have been published.
How do such models work? How can we be sure they are accurate? What do they tell us?
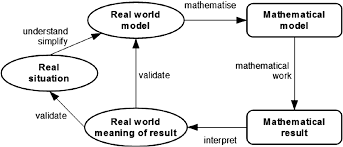
The headline findings from the modelling are the ones that have been delivered to us consistently in governments’ media conferences and other information activities:
An uncontrolled COVID-19 epidemic would result in a situation dramatically exceeding the capacity of the Australian health system over a prolonged period, notwithstanding the increases in that capacity that are possible.
A combination of case-targeted isolation measures with general social measures will substantially reduce transmission and result in a more prolonged epidemic with lower peak incidence, fewer overall infections and fewer deaths.
As we all know, we have to stay home.
How it works
These general prescriptions from the modelling are clear and largely unchallenged. But as time passes it will be good if there is closer scrutiny of this and other modelling. This will result in better understanding of both the general applicability of such modelling and the specific work being done on the Australian government’s preferred model.
The key variables on which mathematical models of infection are based are the latent period (i.e. the interval following exposure before an individual becomes infectious and transmits the disease), the infectious period (i.e. the period during which an infected person can transmit a pathogen to a susceptible host), and transmissibility. Transmissibility is described by the reproduction number – the number of secondary cases generated by a single infected case introduced into a susceptible population.
If the transmissibility number is less than 1, infection is receding. If it’s greater than 1, infection is spreading.
For models of this kind it is useful to know the extent to which outputs (in effect, the model’s predictions) change in response to a given amount of variation in its inputs, and the particular input to which altered outputs can be attributed. The inputs include both the assumptions made about the structure of the entity being modelled and the data fed in.
This is the business of uncertainty and sensitivity analysis. In effect they provide information about the robustness of the model – the probability of the model and its predictions being accurate reflections of reality. The greater the model’s uncertainty or sensitivity, the more its outputs change with a given amount of variation of its inputs – and the less useful it will be.
Such analyses can help check the accuracy of a model’s structure or specification by assessing the individual contribution of a variable and the need to include it or not.
They can also help interpret the results of a model by identifying thresholds for certain variables that trigger outcomes of interest.
The value of any such modelling is limited if the model’s structure is imperfect (that is, if it makes false assumptions about the relationships between elements of the model) or if incomplete or inaccurate data are fed into it. The modelling can be run again and again with greater confidence about its accuracy as, each time, more is known about the characteristics of thepathogen and more local (Australian) data are added in.
Critically, accurate estimation of the transmissibility of a disease requires reliable data on its incidence in the total population. As we have been told time and time again, this requires “testing, testing and testing”.
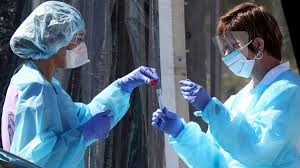
In addition to the latent period, the infectious period and transmissibility, more specific variables can be included in the model, such as the structure of the population and its mobility patterns, demographic variables, risk factors and age profiles. But with every new variable included the risk of false assumptions or imperfect data is likely to increase.
The preferred Australian model for use with COVID-19
There are a number of mathematical models doing their stuff around the world on the spread of infectious diseases and the impact of various public health responses. The one that has been, and remains, the basis for the decisions of Australian governments on the COVID-19 crisis is managed by the Peter Doherty Institute for Infection and Immunity, a joint collaboration of the University of Melbourne and the Royal Melbourne Hospital.
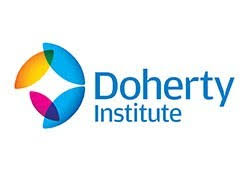
The Doherty team released to the public a paper on 7 April 2020 about their modelling work on COVID-19.
The paper is quite open about potential weaknesses of the model that stem from unavoidable uncertainties about the assumptions made and imperfect or incomplete data relating specifically to the progress of the disease in Australia. Since it is a new pathogen there are uncertainties about the true disease ‘pyramid’ for COVID-19, and a lack of information about determinants of severe (as distinct from mild) disease. In the modelling done so far, age has been used as a best proxy for the probability of symptoms becoming severe.
There are other uncertainties. The model being used has been converted from one used for influenza and there are great differences between that pathogen and COVID-19. The assumptions about reducing transmission of influenza through a combination of distancing measures come not from Australian data but from Hong Kong. The relative contributions of different measures, such as the cancellation of mass gatherings, distance working, closure of schools or cessation of non-essential services, are not yet clear.
More will be learned about these particular strategies from real time data now being collected by various Australian agencies. In turn this will enable the more precise estimation of transmissibility for COVID-19 in Australia. This will be used to update forecast trajectories of the epidemic. These will no doubt be among the key pieces of information used by governments to manage the ongoing responses to the pandemic.
Some critical unknowns
Perhaps the most serious and alarming reminder in the published paper is that low and middle-income countries will find it even harder – potentially quite impossible – to deal with the COVID-19 crisis. Their health systems are already weaker, with limited access to high level care.
Given the massive impact on world trade and damage to the budgets of all countries, including those that are affluent that have been donors of international aid, the impact of the COVID-19 emergency on the people and governments of poorer countries may become quite unmanageable.
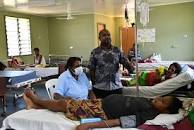
Much will depend on the role played by international aid and trade in the new order.
One of the most critical omissions from Australia’s modelling to date is that it has not as yet accounted for the loss of health care workers due to illness, carer responsibilities or burnout. Nor has it accounted for shortages of critical medical supplies, because the true extent of these shortages and their likely future impacts on service provision are apparently still unknown.
These are two aspects of the issue to which governments and others must continue to give urgent attention. Apart from anything else it reminds us of the very special place of health care workers and the risks they face. Let’s continue to applaud, thank and support them.
Uncertainty and sensitivity analyses may perhaps already have been used by the team in Melbourne to investigate any number of aspects of the modelling. For example the published paper reports that in the simulation of a case-targeted public health intervention it was assumed that 80% of the identified contacts adhered to quarantine measures. It would be useful to know how many additional ICU beds would be required if the compliance rate was 78% or 82%, for example.
With the publication of the Institute’s paper a start has been made down the track of ensuring that the public becomes more familiar with, and more trusting of, the basis of many of the decisions affecting them. It will help to equip the Australian people for the kinds of generous and determined responses that will be needed for global recovery.